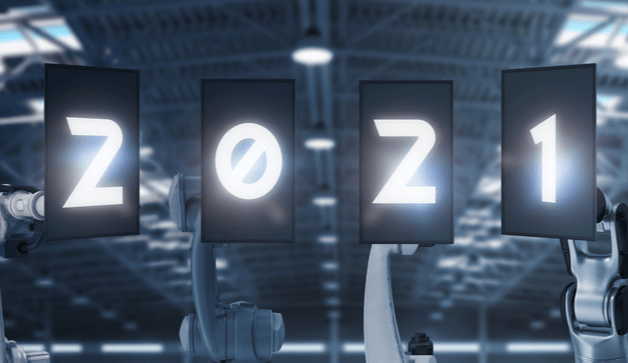
Machine learning is an exciting and critical technology that has continued to increase in popularity over the past several years. Today, the odds that you have come across the popularity of machine learning and artificial intelligence are pretty high, especially if you work in a heavily technologically advanced industry.
Machine learning is being integrated into more organizations, including Google and Netflix. Even smaller organizations are implementing machine learning algorithms to derive valuable insights from their data as well.
According to Market Research, the global machine learning market will grow from $7.3 billion in 2020 to $30.6 billion in 2024. This growth signifies a progressive trend throughout 2021 and beyond.
A Salesforce Research survey notes that 83% of IT experts are convinced artificial intelligence and machine learning are transforming the customer engagement journey. This perspective significantly shows that machine learning is only going to become more popular and more useful.
Key Machine Learning Trends in 2021
As we look forward to a productive new decade, it is important to examine some of the relevant machine learning trends that have the potential to transform the future for the better. Keep in mind that these trends reveal not only the experimentation of machine learning in several industries but also significant development and progress in the technology itself.
-
Machine learning in hyper-automation
Hyper-automation, which Gartner has identified as an IT mega-trend, is the likeliness that virtually everything in an organization that can be automated. Legacy business procedures, for example, should actually be automated. The COVID-19 crisis has significantly increased the adoption of this phenomenon, which is otherwise known as intelligent process automation and digital process automation.
Machine learning and AI are crucial aspects and indispensable propellers of hyper-automation (in addition to various innovations such as process automation tools). For the sake of effectiveness, hyper-automation processes cannot rely on statically packaged software. Automated enterprise processes must have had the ability to adapt to evolving conditions and respond to unexpected situations.
-
Business analysis and predictions
This strategy allows experts to collect and analyze a set of data over a time frame that is thereafter screened and used to make smart decisions. Machine learning networks can provide suppositions with accuracy as much as about 95% whenever trained using multiple data sets.
In 2021 and beyond, we can expect that companies should integrate recurrent neural networks for high-fidelity prediction. For instance, machine learning solutions can be integrated to unravel hidden trends and precise predictions. A clear illustration of this can be seen in insurance companies identifying likely frauds that could in one way or another have great impact on them.
(Also Read: What is Machine Learning?)
-
Automated machine learning
The next phase of growth in machine learning trends is automated machine learning. It is a good idea for individuals who are not professionals in the complex world of machine learning as well as for professional data analysts and scientists. Automated machine learning enables these data experts to build machine learning models with increased productivity and efficiency while featuring extremely high quality.
Thus a tool such as AutoML can be utilized to train top-quality custom ML models for clustering, regression, and classification without extensive knowledge of how to program. It can seamlessly produce an adequate level of customization without an in-depth understanding of the complicated workflow of machine learning. It can also be useful in utilizing machine learning best practices while saving resources and time.
A good example of such AutoML is automated machine learning created by Microsoft Azure which can design and launch predictive models.
-
Synchronization of machine learning and IoT
The Internet of Things is already a developed technology that involves the connection of several “things” or devices across a network, each having the ability to interact with each other. These devices are continually increasing, at such a rate that there is the possibility of having over 64 billion IoT devices by the end of 2025.
The function of all of these devices is to collect data that can be evaluated and processed to generate useful insights. This is where ML becomes very pertinent. ML algorithms can be utilized to transform the data gathered by IoT devices into useful actionable outcomes.
A good example of this is Green Horizons, which is a project launched by IBM’s Research Lab in China whose goal is to regulate the pollution rates to better breathable levels. This is achievable with the use of an IoT network wherein sensors gather emissions from automobiles, traffic levels, weather, airflow direction, and pollen levels, among other things. Then machine learning algorithms are employed to figure out the most effective way to minimize these emissions.
The synchronization of machine learning and IoT can as well be observed in the area of smart cars where autonomous-driving vehicles must be very precise and each part must communicate with one another in split seconds on the road. This demonstrates how essential the integration of these technologies is.
In fact, Gartner forecasts that over 80% of business IoT projects will utilize AI and ML in one way or the other by 2022. This paced growth is a lot greater than the 10% of enterprise projects currently utilizing it.
-
Faster computing power
AI analysts are basically close to the beginning of understanding the field of artificial neural networks and the most suitable approach to arranging them. This suggests that within the next year, algorithmic successes will continue increasing at an overwhelming pace with pragmatic progress and better problem-solving mechanisms.
Similarly, cloud ML solutions are adding momentum as third-party cloud service platforms support the deployment of machine learning algorithms in the cloud. AI can resolve a reasonable range of unfavorable issues that require discovering insights and making decisions. Although, in the absence of the ability to lay hands on a machine’s proposition, people will assume that it is cumbersome to accept that suggestion. With defined lines, conceive continued development in the transitional period increasing the explainability and transparency regarding Artificial Intelligence algorithms.
-
Reinforcement learning
Reinforcement Learning (RL) is also one of the machine learning trends to look out for this year. It can generally be used by businesses in the near future. It is an innovative use of deep learning which makes use of its personal experiences to enhance the effectiveness of accumulated data.
In RL, Artificial Intelligence programming is deployed with several conditions that determine what kind of activity will be executed by the software. In respect to various actions and outcomes, the software auto-learns actions to work to achieve the proper ultimate goal.
A typical illustration of RL is a chatbot that attends to basic user queries such as consultation calls, order booking, greetings. ML Development Organizations can make use of Reinforcement Learning to ensure the ingenuity of the chatbot by including sequential conditions to it – for instance, differentiating prospective buyers and transferring calls to the appropriate service agent. Some other applications of reinforcement Learning are aircraft control, industrial automation, robot motion control, as well as business processes and strategic planning.
-
Machine learning in cybersecurity
As the popularity of machine learning continues to skyrocket in this contemporary time, it is likewise spreading its applications in several various sectors. One of the most common among these industries is the cybersecurity industry. Machine learning has a lot of applications in cybersecurity, some of which are detection of cyber threats, combating cyber-crime that equally utilizes ML capabilities, enhancing available antivirus software, among other things.
Machine learning is also employed in the creation of smart antivirus software that can detect any malware or virus by its irregular behavior instead of just utilizing its signature like regular antivirus. Hence, the smart antivirus has the capacity to detect older threats from formerly experienced viruses and also fresh threats from viruses that were newly created by screening their abnormal behavior. Several companies are integrating machine learning into cybersecurity. Some of the most popular examples are Sqrrl, a firm established by ex-National Security Agency employees and Chronicle, a cybersecurity organization managed by Google’s parent company, Alphabet.
Final Thoughts
In this new decade, we anticipate a lot of new machine learning trends to be observed in different industries. Indeed, last year was one year no one could have predicted (at least not in a good way!). So no one can really say what the rest of this year really holds. Nonetheless, it is conspicuous that a couple of trends in machine learning are going nowhere, like its fusion into a lot of new fields including healthcare, finance, and cybersecurity.
An increasing number of businesses are embracing machine learning so that this innovation is no longer restricted to only tech giants such as Microsoft, Facebook, and Google. This means that artificial intelligence ethics is becoming pertinent as many organizations develop and expand their ethics committees.